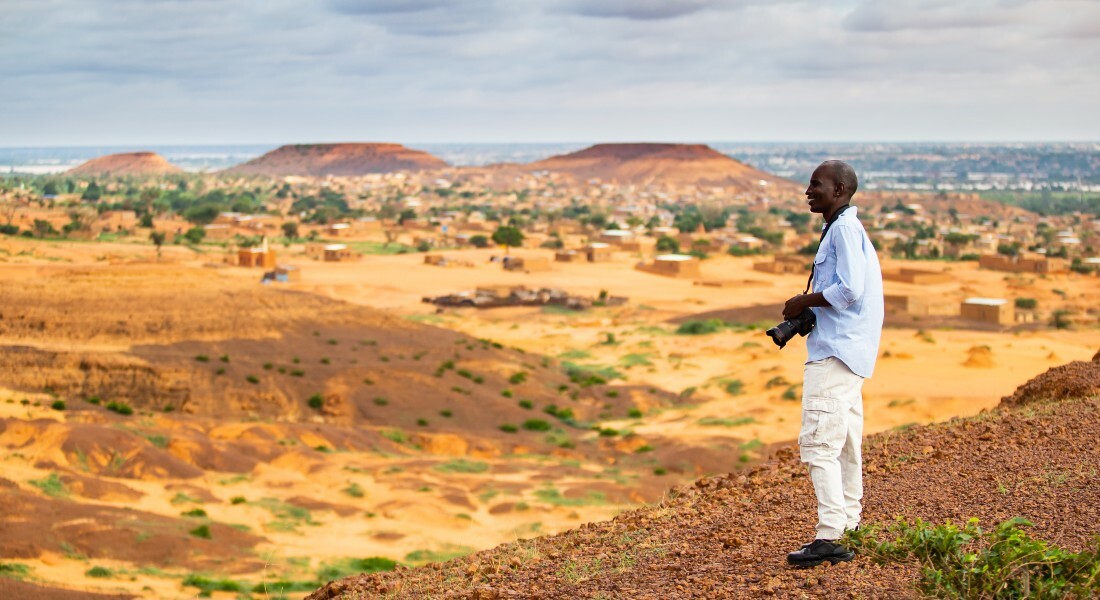
Department of Computer Science
DeReEco will combine remote sensing and artificial intelligence technologies to monitor, predict, and simulate changes in ecosystem properties – such as human settlement structures, agricultural use, tree and forest cover, water bodies, and carbon stocks – at a global scale.
Based on newest remote sensing imagery, novel machine learning models will be developed to understand the complex relationships between temporal dynamics in geospatial datasets. The knowledge gained on status, dynamics, and drivers of ecosystem changes will be pivotal in land degradation assessment (e.g., deforestation), in mitigating poverty (e.g., food security, agroforestry, wood products), and in managing climate change (e.g., carbon sequestration).
Climate change and population growth are currently reshaping global ecosystems at an accelerating rate causing significant changes in global environmental resources. Land degradation, which causes losses in productivity, carbon storage, and biodiversity, is said to aggravate sustainable livelihoods worldwide, and adverse impacts from extreme climate events are expected to become more frequent. Deforestation plays an important role in the global carbon budget and for changes in the global atmospheric CO2 concentration.
Our knowledge of the current state of ecosystem resources and associated services supporting human society (e.g., food, fresh water, and wood) is still deficient, and also the drivers of ecosystem changes are not well understood, thereby impeding efficient adaptation and mitigation strategies to be implemented. Remote sensing remains the only viable tool to map and monitor our ecosystem resources at a global scale and an ever-increasing stream of satellite data holds great potential for unravelling these major unknowns for improved sustainable resource management.
Yet, our ability to effectively make use of this wealth of different satellite technologies for an improved understanding of global change is currently hampered by insufficient methods for handling and analysing the large amounts of spatio-temporal environmental data available.
Deep learning has been the main driver of the tremendous progress in artificial intelligence (AI) over the last decade and it holds great promise for remote sensing and Earth system sciences as well as for tackling climate change. However, methodological challenges have to be met to exploit the full potential of deep learning in remote sensing of the environment. Novel deep learning approaches have to be developed, which (i) monitor changes robustly and under changing input distributions, (ii) accurately detect and count individual objects, (iii) process multi-channel spatio-temporal information, and (iv) can be coupled with physical models – and all this efficiently such that petabytes of data can be processed in the context of global-scale ecosystem analyses.
The inter-disciplinary design of DeReEco addresses these challenges to redefine our current understanding of global ecosystem functioning and dynamics. DeReEco will advance both remote sensing as well as AI by devising conceptually new systems that can be used to better monitor ecosystem related changes and to predict and simulate the influence of climate change and human intervention on our ecosystem at a global scale.
The DeReEco team has already made promising initial steps in this direction by developing a deep learning system that accurately quantifies individual trees in regions of Africa with immediate implications for our understanding of the role of woody resources in coupled human-environmental systems.
The techniques and systems produced by DeReEco will pave the road for new research fields targeting an improved understanding of the climate-human-environment nexus. By coupling data on vegetation ecosystem services, human management and climate change with geospatial longitudinal data on human consumption/needs (food and fodder supply), a better understanding of resource distribution in time and space can be achieved. Such information will ultimately be used to quantify linkages between ecosystem conditions and health state for an improved understanding of food security.
DeReEco is supported by NASA and the Danish Meteorological Institute.
The project is funded by The Villum Foundation.
Name | Title | Phone | |
---|---|---|---|
Ankit Kariryaa | Assistant Professor - Tenure Track | +4535328046 | |
Christian Igel | Professor | +4535335674 | |
Christin Abel | Assistant Professor | +4535332467 | |
Fabian Christian Gieseke | Associate Professor | ||
Gyula Mate Kovács | Postdoc | +4591922293 | |
Hui Zhang | PhD Fellow | +4535327586 | |
Nico Lang | Postdoc | +4535323911 | |
Rasmus Fensholt | Professor | +4535322526 | |
Stefan Oehmcke | Assistant Professor | ||
Stéphanie Horion | Associate Professor | +4535325878 | |
Venkanna Babu Guthula | PhD Fellow | +4535328915 | |
Xiaoye Tong | Assistant Professor | +4535331239 |
Rasmus Fensholdt, Department of Geosciences and Natural Resource Management
Christian Igel, Department of Computer Science
Project: Deep Learning and Remote Sensing for Unlocking Global Ecosystem Resource Dynamics (DeReEco)
Period: 2020-2025
Funding: DKK 14.9 million