New AI project will take road detection from manual to automatic
The Microsoft AI for Earth program, working to solve global environmental challenges, is supporting the new project Monitoring Global Changes via Distributed Machine Learning at the Department of Computer Science, University of Copenhagen (DIKU).
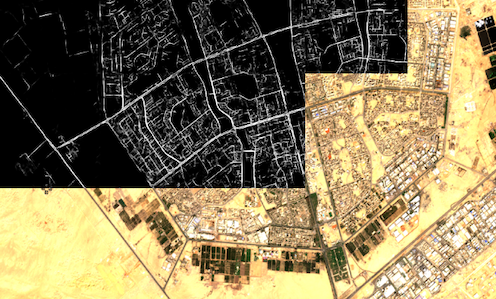
The aim of the project is to create and implement an automatic system for detecting objects or infrastructure (e.g. roads) based on satellite data as well as for monitoring their changes at global scale. The system will be based on state-of-the-art machine learning models and distributed computing frameworks, enabling it to handle data volumes in the terabyte and petabyte range. It is expected to be of great value for a variety of application cases such as an accurate and up-to-date mapping of georeferenced roads which is vital to a great deal of applications such as GPS systems, urban planning, monitoring climate change, detecting infrastructure for crisis management and more. The image illustrates the output of fully automated georeferenced road predictions from satellite data.
Surprisingly, such detection tasks are still mostly done in a manual fashion by visual inspection of satellite data or with limited tool support, which can quickly become very time-consuming. This approach is clearly not suited for processing huge amounts of satellite data covering large regions of our Earth, which now has become available for free.
Recent advancements in high-resolution imaging satellites have fueled the emergence of a wide variety of remote sensing applications. The high-resolution images allow the identification of fine details in the landscape and recent breakthroughs in machine learning dramatically advance the extraction of high-level information from such images. These advancements is what has now made this new project possible.
Contact
Fabian Gieseke
fabian.gieseke@di.ku.dk
Assistant Professor, Department of Computer Science, University of Copenhagen
Tina Virenfeldt Kristensen
tikr@di.ku.dk
Communication Consultant, Department of Computer Science, University of Copenhagen
About AI for Earth
AI for Earth puts Microsoft cloud and AI tools in the hands of those working to solve global environmental challenges. Through grants that provide access to cloud and AI tools, opportunities for education and training on AI, and investments in innovative, scalable solutions, AI for Earth works to advance sustainability across the globe.
Project Members
- Andreas Borgstad (MSc Student)
- Fabian Gieseke (Assistant Professor)
- Wouter Kouw (Postdoc)
- Marcos Vaz Salles (Associate Professor)
- Christoffer Thrysøe (MSc Student)