AI Seminar: Safe Testing
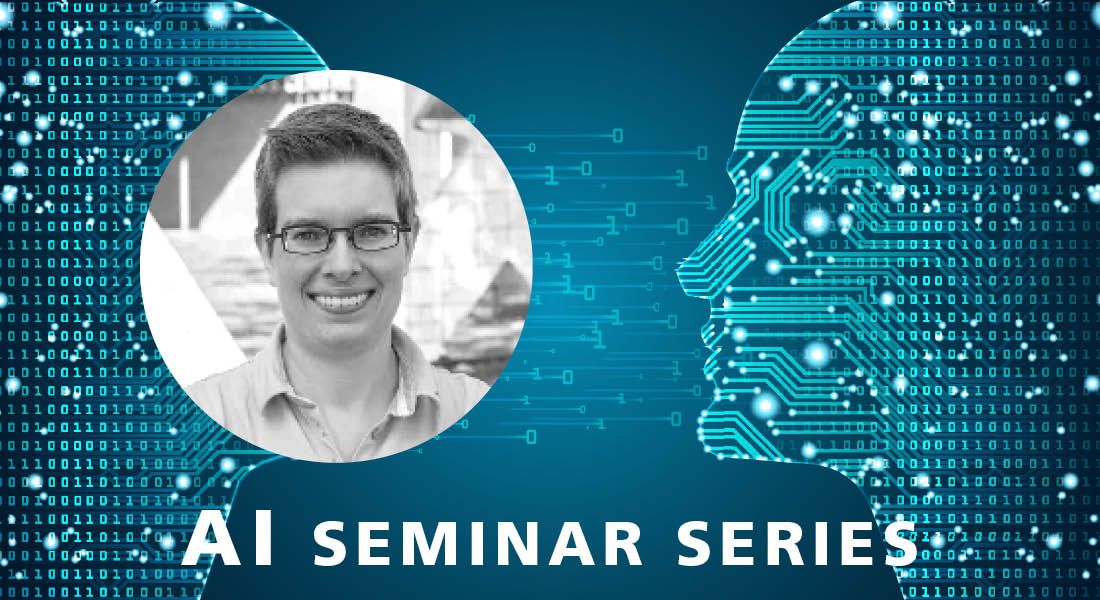
Livestream
The event will be livestream over zoom (https://ucph-ku.zoom.us/j/69078696930?pwd=K2tJNHczcU1QbGVqYllFdFhDcmlLQT09), for those of you would rather participate remotely.
Speaker
Rianne de Heide, PhD Candidate, Leiden University.
Abstract
We present a new theory of hypothesis testing. The main concept is the E-value, a notion of evidence which, unlike p-values, allows for effortlessly combining evidence from several tests, even in the common scenario where the decision to perform a new test depends on the previous test outcome: safe tests based on E-values generally preserve Type-I error guarantees under such "optional continuation". E-values exist for completely general testing problems with composite null and alternatives. Their prime interpretation is in terms of gambling or investing, each E-value corresponding to a particular investment. Surprisingly, optimal "GROW" E-values, which lead to fastest capital growth, are fully characterized by the joint information projection (JIPr) between the set of all Bayes marginal distributions on H0 and H1. Thus, optimal E-values also have an interpretation as Bayes factors, with priors given by the JIPr. We illustrate the theory using two classical testing scenarios: the one-sample t-test and the 2x2 contingency table. In the t-test setting, GROW s-values correspond to adopting the right Haar prior on the variance, like in Jeffreys' Bayesian t-test. However, unlike Jeffreys', the "default" safe t-test puts a discrete 2-point prior on the effect size, leading to better behavior in terms of statistical power. Sharing Fisherian, Neymanian and Jeffreys-Bayesian interpretations, E-values and safe tests may provide a methodology acceptable to adherents of all three schools.
This seminar is a part of the AI Seminar Series organised by SCIENCE AI Centre. The series highlights advances and challenges in research within Machine Learning, Data Science, and AI. Like the AI Centre itself, the seminar series has a broad scope, covering both new methodological contributions, ground-breaking applications, and impacts on society.