AI Seminar: Interpreting Training
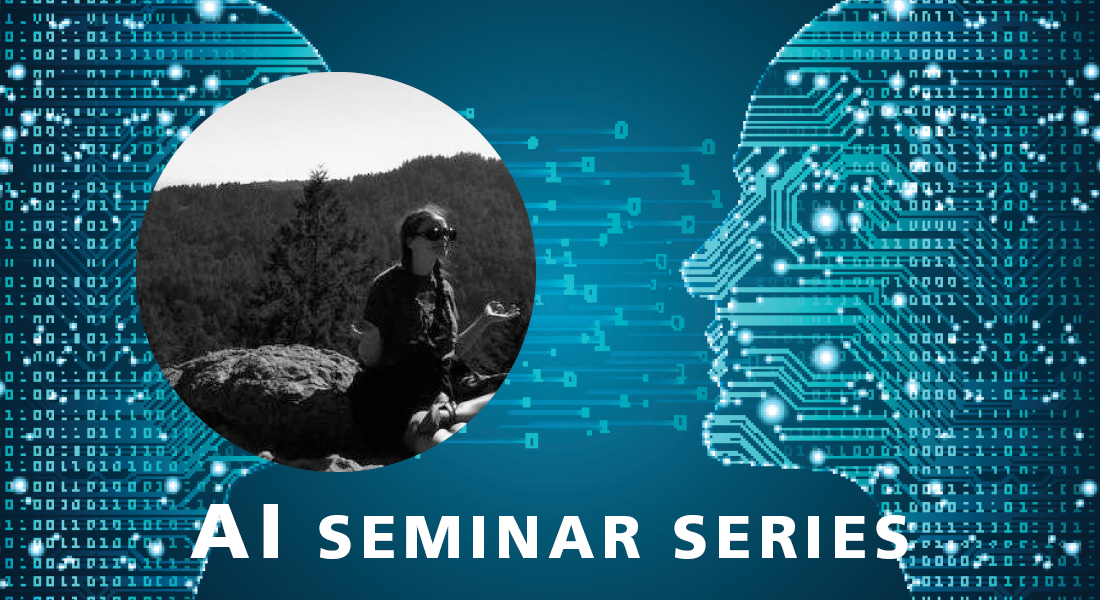
Join us for a talk by Naomi Saphra, Postdoctoral Researcher at New York University. Everybody is welcome to attend.
Title
Interpreting Training
Abstract
Interpretability research in NLP often follows a predictable pattern—pick an indicator of structure or knowledge such as probe or challenge set accuracy, measure that indicator in a fully trained model, and assert that this structure or information is integral to how the model functions. However, we can achieve a much deeper understanding by considering how these indicators emerge from the training process. First, this talk will discuss research on the relationship between interpretable generalization behavior and the presence of multiple basins on the loss landscapes of finetuned text classifiers. Then, I will describe how manipulating interpretable behaviors during the training process can shed light on the role of syntactic signals in attention distributions and generally on simplicity bias both helps and hurts language model performance. These results form the basis of a manifesto for exploring developmental explanations when researching interpretability and generalization behavior.
Bio
Naomi Saphra is a postdoctoral researcher at NYU with Kyunghyun Cho. She is interested in NLP training dynamics: how models learn to encode linguistic patterns or other structure and how we can encode useful inductive biases into the training process. Previously, she earned a PhD from the University of Edinburgh on Training Dynamics of Neural Language Models, worked at Google and Facebook, and attended Johns Hopkins and Carnegie Mellon University. Outside of research, she plays roller derby under the name Gaussian Retribution, does standup comedy, and shepherds disabled programmers into the world of code dictation.
Anyone can participate in the event, but please use this form to register.
This seminar is a part of the AI Seminar Series organised by SCIENCE AI Centre. The series highlights advances and challenges in research within Machine Learning, Data Science, and AI. Like the AI Centre itself, the seminar series has a broad scope, covering both new methodological contributions, ground-breaking applications, and impacts on society.