AI Seminar: Machine Learning for Earth System Science
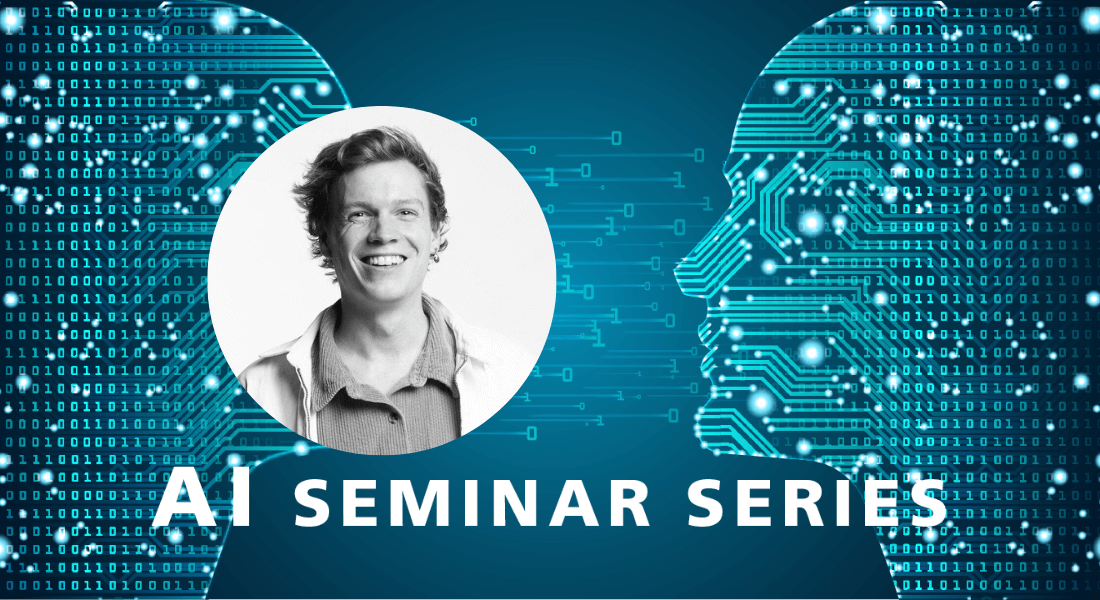
Join us for a talk by Björn Lütjens, postdoctoral associate in the MIT Department of Earth, Atmospheric, and Planetary Sciences. Everybody is welcome to attend.
Title
Machine Learning for Earth System Science
Abstract
Projections of climate change from full-complexity Earth system models (ESMs) are essential for climate policy, but computationally very expensive. As a result, ESMs have only been run for a few representative assumptions on future greenhouse gas and aerosol emissions, such as CMIP6 ScenarioMIP. More efficient emulators are used for climate impact studies that require custom emission pathways, such as sensitivity analysis, but often take simplifying assumptions that break down for simulating nonlinear aerosol impacts, extreme statistics, or negative emission pathways. In this talk, I will share how novel machine learning (ML) methods can be adapted towards overcoming these computational difficulties in climate modeling. In particular, I will show that a 100M-parameter foundation model can be outperformed by a simple linear regression model on the most cited climate emulation benchmark, when leveraging existing knowledge from the climate sciences. In this talk, I will also introduce a novel integration of a physics-based model into a geospatial generative vision model. More generally, I will share how combinations of ML with physics-based models are fundamental to driving meaningful progress in Earth system science.
Bio
Dr. Björn Lütjens is a postdoctoral associate in the MIT Department of Earth, Atmospheric, and Planetary Sciences, where he develops advanced machine learning approaches to reduce the computational cost of Earth system models, together with Prof. Raffaele Ferrari and Prof. Noelle Selin. His research is part of the MIT Climate Grand Challenge BC3 and has won grants from big tech (IBM), government (NSF), nonprofit (CCAI), and space (ESA) sectors. He has earned a Ph.D. from MIT with Prof. Dava Newman in machine learning and earth system modeling, an M.Sc. from MIT in safe and robust deep reinforcement learning, and a B.Sc. from Technical University of Munich in Engineering Sciences.
This seminar is a part of the AI Seminar Series organised by SCIENCE AI Centre. The series highlights advances and challenges in research within Machine Learning, Data Science, and AI. Like the AI Centre itself, the seminar series has a broad scope, covering both new methodological contributions, ground-breaking applications, and impacts on society.