AI Seminar: Mysteries in multi-task learning and input representations for language models
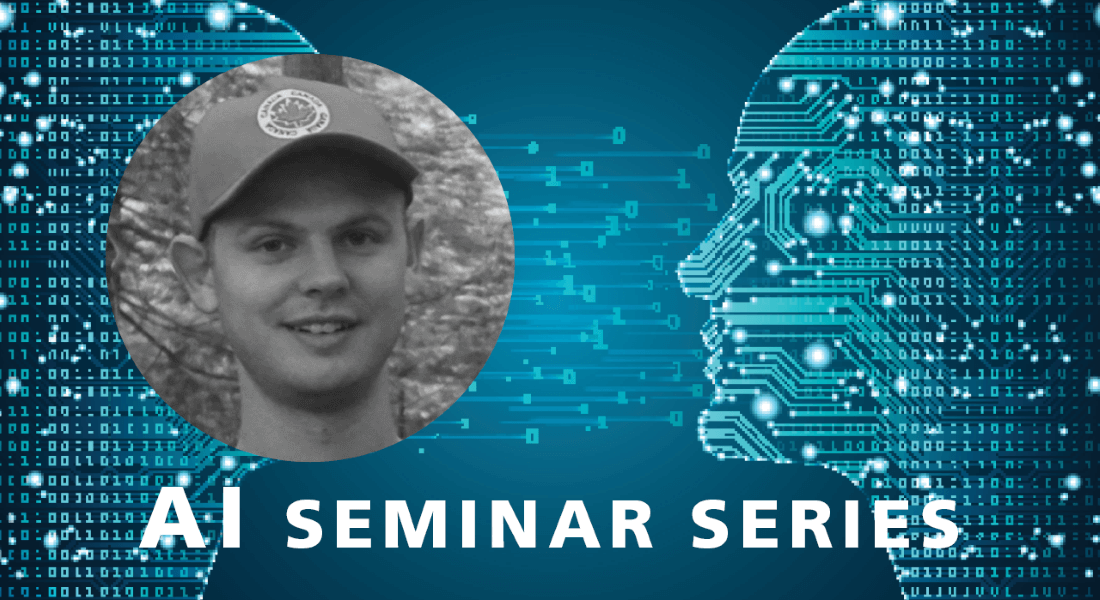
Abstract:
Natural Language Processing (NLP) conventionally focuses on modeling words, phrases, or documents. However, natural language itself is data generated by people. With the growth of social media and automated assistants, NLP is increasingly tackling human problems that are social or psychological in nature. Further, traditional NLP task research is realizing pitfalls from a lack of accounting for social contexts, such as disparities in predictive outcomes or model accuracy. In this talk, I will present work towards a vision of a human-centered NLP which begins to account for human and social context within modeling approaches. This includes controlling for and correcting biases from extralinguistic variables, using ecologically valid language models, placing language in time, and leveraging the inherent multi-level structure (sequences of words are generated by people, who in turn belong to communities). Taken together, I will suggest that considering the people behind the language not only offers opportunities for improved accuracy but it could be fundamental to NLP's role in our increasingly digital world.
Bio:
Rob van der Goot's main interest is in low-resource setups in natural language processing, which could be in a variety of dimensions: including language(-variety), domain, or task. He is currently trying to use multi-task learning in these setups to reduce the dependence on training data. His PhD was on the use of normalization for syntactic parsing of social media data, one specific case of a challenging transfer setup.
This seminar is a part of the AI Seminar Series organised by SCIENCE AI Centre. The series highlights advances and challenges in research within Machine Learning, Data Science, and AI. Like the AI Centre itself, the seminar series has a broad scope, covering both new methodological contributions, ground-breaking applications, and impacts on society.